
Future Data Scientist

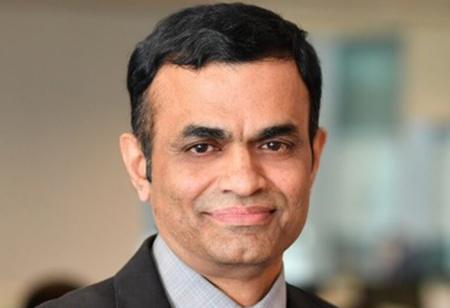
Ram Kumar, Chief Data and Analytics Officer, Cigna, 0
Is Data Science still the sexist job of the 21st century?
In 2012, data science was described as the 'Sexiest job of the 21st century' by Harvard Business Review. The role of datascientist has become an increasingly critical one for businesses, uncovering patterns and insights from business data that help businesses stay competitive by allowing them to quickly respond to trends, and customer and, partner needs, and make informed decisions. With the advancements in the field of Artificial Intelligence (AI) and Machine Learning, the role of data science to support these fields is critical and therefore, data scientist profession will remain a sexy profession for a long time. The field of data science is changing rapidly and it is important for the data scientists to be on top of these changes to adapt themselves to compete in the future.
My Prediction
My prediction is that in the next few years, data scientists will be everywhere and in abundant numbers and will be the norm like what happened to software engineering field in the early 1990s which saw a significant influx of software engineers everywhere. The evolution of 4th generation language in the field of Software Engineering in the 1990s led to a number of people entering the field of software engineering without the need to have any background in computer science which required deep understanding of data structures and algorithms which is the core component of software engineering. 4th generation software development tools were introduced which led to interactive and integrated software development tools that assisted in generating programming codes automatically. Programming languages such as Java were introduced with automatics memory management capability irrespective of the underlying operating systems unlike programming languages such as Pascal and C.
Similarly, data science will go through a change where one would not need to understand about analytical, machine learning and deep learning models and algorithms as industry domain specific models would have been pre-built for data scientists to choose from and build upon it using an integrated analytical model development environment by just focusing on the right data and variables to feed to the models. Therefore, the
current excitement among data scientists to build analytical models such as predictive and deep learning models using languages like Python by applying several algorithmic techniques will fade away in the future. It will become the norm.There will be an increasing shift towards more self-service capabilities in data science and AI with next generation data intelligence tools, and therefore, the pool of people generating data driven business insights will expand beyond just analysts and data scientists.
This new breed of data tools will make it easier for people across business functions to access and explore the data on their own to generate insights. As a result, we’re going to see an unprecedented number of insights being generated within companies than ever before. But that is not enough to generate true business value out of data.
Key Skills Required for Data Scientist
So, let me discuss about the key skills required for a data scientist to be competitive in the future:
•Data Lifecycle. Data is the life blood of Data Science and AI and therefore, it is critical that every data users/data consumers’ responsibility to respect and treat data as a key strategic asset of the organisation and ensure the asset is managed effectively and efficiently to create appreciable value out of it. It is therefore, important for a data scientist to have a deep understanding of the lifecycle of data used for analytics and not just about understanding data preparation and data analysis/analytics only which is just the “use” component of the data lifecycle. When you ask the current data scientists to explain what a lifecycle of data means, majority of them have no clue despite using data day in day out. Lifecycle of data covers collection, organisation, storage, use and retention/destruction of data and is supported by data quality, metadata, master data, data lineage, data privacy, data security, data ethics, data governance and data risk management.
•Business Subject Domain Expertise. Deep understanding of the business subject domain is critical for a data scientist to build the right data driven data science solutions and importantly, the ability to operationalise the data science outcomes in business environment for effective and efficient use and, monitor and measure sustainable business value generated.
•Data Story Telling. Unless data scientists can improve the communication of business insights generated through descriptive, predictive analytics or prescriptive analytics to the business community in an easily understandable business subject domain language, we will also see a poorer insight-to-value conversion rate. If an insight isn’t understood and isn’t compelling, no one will act on it and no change will occur. Numbers have an important story to tell. They rely on you to give business stakeholders a clear and convincing voice. Any insight worth sharing is probably best shared as a “data story”. The phrase “data storytelling” has been associated with many things such as data visualizations, data presentations, dashboards, and infographics. Too often data storytelling is interpreted as just visualizing data effectively, however, it is much more than just creating visually appealing data charts. It is a structured approach for communicating data insights, and it involves a combination of three key elements: data, visuals, and narrative. Data story telling is therefore, a critical skill that a data scientist must possess.
This new breed of data tools will make it easier for people across business functions to access and explore the data on their own to generate insights. As a result, we’re going to see an unprecedented number of insights being generated within companies than ever before. But that is not enough to generate true business value out of data.
Key Skills Required for Data Scientist
So, let me discuss about the key skills required for a data scientist to be competitive in the future:
•Data Lifecycle. Data is the life blood of Data Science and AI and therefore, it is critical that every data users/data consumers’ responsibility to respect and treat data as a key strategic asset of the organisation and ensure the asset is managed effectively and efficiently to create appreciable value out of it. It is therefore, important for a data scientist to have a deep understanding of the lifecycle of data used for analytics and not just about understanding data preparation and data analysis/analytics only which is just the “use” component of the data lifecycle. When you ask the current data scientists to explain what a lifecycle of data means, majority of them have no clue despite using data day in day out. Lifecycle of data covers collection, organisation, storage, use and retention/destruction of data and is supported by data quality, metadata, master data, data lineage, data privacy, data security, data ethics, data governance and data risk management.
•Business Subject Domain Expertise. Deep understanding of the business subject domain is critical for a data scientist to build the right data driven data science solutions and importantly, the ability to operationalise the data science outcomes in business environment for effective and efficient use and, monitor and measure sustainable business value generated.
•Data Story Telling. Unless data scientists can improve the communication of business insights generated through descriptive, predictive analytics or prescriptive analytics to the business community in an easily understandable business subject domain language, we will also see a poorer insight-to-value conversion rate. If an insight isn’t understood and isn’t compelling, no one will act on it and no change will occur. Numbers have an important story to tell. They rely on you to give business stakeholders a clear and convincing voice. Any insight worth sharing is probably best shared as a “data story”. The phrase “data storytelling” has been associated with many things such as data visualizations, data presentations, dashboards, and infographics. Too often data storytelling is interpreted as just visualizing data effectively, however, it is much more than just creating visually appealing data charts. It is a structured approach for communicating data insights, and it involves a combination of three key elements: data, visuals, and narrative. Data story telling is therefore, a critical skill that a data scientist must possess.