
BI & Data Science: Complementing Each Other To Drive Data Driven Value Creation For An Organization

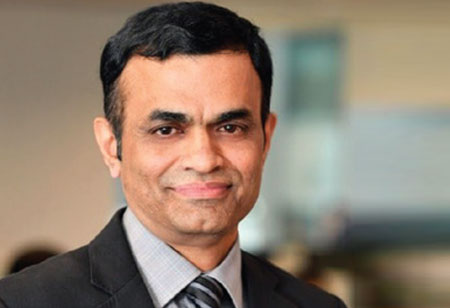
Ram Kumar, Chief Data & Analytics Officer, Cigna, 0
There is no universally accepted definition for Business Intelligence (BI). Business Intelligence is a term that could mean different things to organizations and people. For some, it may be just a set of basic Key Performance Indicator (KPI) reporting or dashboard generated using data about what is happening to the business; for others, it may be insights generated out of the data sets using statistical techniques that cannot be seen through naked eye or analysed manually by human brain and for some, it may be about predicting/forecasting the like lihood of the performance of the business across a set of KPIs in the future by using advanced analytical method/ techniques. But regardless of methods or tools used and, in my view, they provide data analysis driven insights/facts for decision making to business stakeholders, according to their requirements and business context. Context here is important because the same data sets that are used to create actionable insights for a particular business function could be used to create different actionable insights for another business function, e.g.,finance and under writing functions in insurance.
If your business does not have a good hold of what is happening to its business performance and why through data driven insights in the form of dash board or operational reports, what is the point in thinking about what will happen to the business by using advanced data driven insights? E.g., data science/machine learning. From a Business function and Process standpoint, there is not much difference between Data Science and Business Intelligence they both support business decision making based on data. Probably, this is why it is often assumed in businesses starting their first Data Science or AI projects, that Data Science is the same old Business Intelligence that works much more cleverly and that a Data Science project can be done on the top of existing BI infrastructure and processes.
It is imperative that you need both BI and Data Science to create appreciable business value out of your data. I therefore, call BI and Data Science the 'Yin' and 'Yang' of data driven value creation for the business as they are complementary to each other and each has a place that will solve different business problems. To put it in simple terms, my view is that Business Intelligence (BI) includes operational reporting & dashboards, descriptive analytics, predictive analytics, prescriptive and disruptive analytics. All these approaches provide some kind of intelligence to the business through data analysis and insights by answering different types of business questions (e.g.known knowns, known unknowns, unknown unknowns) and expectations. This is achieved with the support of various techno logies, tools, methodologies and techniques e.g., Visualization tools, R, Hadoop, SQL, Python, Tensorflow, Machine Learning, Deep Learning, intelligent automation, AI solution, and infra structure to handle different types of data assets of varying volume, velocity and veracity. One size approach to create a data storage and processing environment to analyze data will not work, as it is a costly exercise and it is important that the data driven value creation strategy and approach should be based on the maturity and capability of the organization, as it is should be viewed as 'holistic data driven cultural transformation journey' by the organization. This means that data assets are the 'crown jewel' of the organization as its strategic and competitive assets and therefore, the journey should be an enterprise effort and not dictated by specific functions like technology and data analytics only.
Fundamental Challenges that Organizations Need to Overcome to Create Value Out of Data
Irrespective of whether an organization uses BI or Data Science or AI (what each means, varies between organizations) to drive data driven digital strategies or intelligent automation or customer experience to drive value creation using data, the challenges that an organization needs to overcome are common. The crucial point is that any organization that invests into these areas without having a solid data foundation and management strategy and practice, will struggle. Any processes or solutions that are built on poor data infra structure and data processes will be slow, untrust worthy, poor quality, resource intensive and expensive. Whether an organization's larger goal is to achieve digital transformation,'compete on analytics' or become 'AI-first', embracing and successfully managing data in all its forms is an essential prereqisite. Critical obstacles with regard to managing data still must be overcome before companies begin to see meaningful benefits from their bigdata, analytics, data science and AI investments.
Whether an organization's larger goal is to achieve digital transformation,'compete on analytics', or become 'AI-first', embracing and successfully managing data in all its forms is an essential prerequisite. Critical obstacles with regard to managing data still must be overcome before companies begin to see meaningful benefits from their big data, analytics, data science and AI investments.
Bringing BI, Data Science & AI Together on a Common 'Data Playground'
How you create a common data layer/infrastructure/ environment that would help derive business value out of data through various initiatives such as BI, Data Science and AI is critical. It is critical for all these initiatives to support each other and work together in a common and consistent well defined environment.
Following are the some of the key initiatives that organizations should consider as part of their holistic data strategy to create the common 'Data playground'.
• Data Democratization: Ensuring that that the organization's data assets flow seamlessly and interoperate across its business processes & technology systems and reaches the hands of the all types of users with minimum fuss with minimum help. This enables business users to seamlessly access data that they can use for value creation. This helps create a datadriven culture throughout the entire organization. Without data democratization, consumers of data waste time searching for data, accessing the data, and waiting for approval. However, it is important to ensure that potential risks such as data ethics, data privacy, and misuse of data & compliance requirements are managed. Data democratization is not a trivial problem, but is fundamental to driving a data driven organization culture. A democratized data environment creates an opportunity for Business intelligence, Data science and AI to work hand-in-hand to derive data driven value to the business in a collaborative, efficient, effective, consistent and reliable manner by seamlessly working with the same data.
•Semantic Data Layer: It is common that data assets of all types in organizations are distributed and silo in nature due to various reasons legacy systems, legacy processes, legacy culture, various servers, public cloud services, and data centres. This has resulted in a massive gap between data sources and business users. Progressive companies are using semantic data layer to help bridge this gap. Semantic Data Layer maps/integrates complex enterprise data assets across the organization to provide a unified, consolidated view of data that would allow for complete and accurate analysis of data.
A semantic layer is a business representation of data. It enables users to discover and access data through selfservice facility without relying on IT, understand the business definition of data (e.g., customer, provider, and products)and its relation ships with other data assets. Even more than that, user could easily start discovering and integrating, on the fly, data from totally different datasets that used different descriptors. Perhaps the greatest achievement of the semantic data layer is to provide different data professionals with easy access to the data needed for their specific roles and tasks. It also provides data lineage and catalogue information about the data assets. The concept of semantic data layer has been in place since the early 1990s. But the significant change in the data landscape due to rise of big data and with increasing push towards data driven digital transfor mation is now making the semantic data layer increasingly important.
Augmented analytics is a key component of the semantic data layer. Augmented analytics uses data science/machine learning, artificial intelligence, and various natural language processing technologies (like natural language generation and natural language query) to enable automation that would accelerate obtaining answers from business data all through a simple, user directed process.
The semantic data layer could be abstracted from the complexities or accessing and querying data from the user by providing a set of Data Service Interfaces (DSI) that could be integrated into business systems whether internal or customer facing and operational processes. With a robust semantic data layer in place, teams can work together more effectively by sharing and collaborating in data value creation, thereby enabling them to derive quality & better insights and make better decisions in less time. Data Science requires close to 80 percent of time in preparing data for value creation, and these overheads can be reduced significantly through the semantic layer. This layer also contributes to managing security and governance of the data assets better. The semantic data layer therefore empowers data processes that democratize data.
•Data Quality:An organization may have the best business and operational processes, the best and state-of-the art technology environment, the smartest brains that can process and analyse data i.e.best data scientists and AI practitioners to generate value, and the best data, technology and digital strategies. But if the underlying data that touches these people, processes and technology is poor in terms of its quality and integrity, the outcomes derived will be poor. Quality of outcomes generated through these initiatives is directly proportional to the quality of data. It is there fore, important that organizations must include 'Data Quality of Design' discipline when planning initiatives at the planning stage itself. Data quality capabilities must include data profiling, cleansing, and enrichment.
•Data Governance:Governing the democratized data, the semantic data layer, the data definitions, data quality, data privacy, data security, data standards, data retention and destruction, and any data related risks is critical. Traditional approaches to data governance where an organization tries to govern all its data assets through a big bang approach rarely works nowadays as businesses neither have the patience nor the budget for it. It is therefore, important to apply smart governance framework by looking at governing the Critical Data Elements that would create value for the business through the use of BI, Data Science and AI, and by incrementally building data governance culture in the organization by demonstrating that data governance enables value creation rather than been seen as controlling or slowing progress.
• Data Democratization: Ensuring that that the organization's data assets flow seamlessly and interoperate across its business processes & technology systems and reaches the hands of the all types of users with minimum fuss with minimum help. This enables business users to seamlessly access data that they can use for value creation. This helps create a datadriven culture throughout the entire organization. Without data democratization, consumers of data waste time searching for data, accessing the data, and waiting for approval. However, it is important to ensure that potential risks such as data ethics, data privacy, and misuse of data & compliance requirements are managed. Data democratization is not a trivial problem, but is fundamental to driving a data driven organization culture. A democratized data environment creates an opportunity for Business intelligence, Data science and AI to work hand-in-hand to derive data driven value to the business in a collaborative, efficient, effective, consistent and reliable manner by seamlessly working with the same data.
•Semantic Data Layer: It is common that data assets of all types in organizations are distributed and silo in nature due to various reasons legacy systems, legacy processes, legacy culture, various servers, public cloud services, and data centres. This has resulted in a massive gap between data sources and business users. Progressive companies are using semantic data layer to help bridge this gap. Semantic Data Layer maps/integrates complex enterprise data assets across the organization to provide a unified, consolidated view of data that would allow for complete and accurate analysis of data.
A democratized data environment creates an opportunityfor business intelligence, data science and AI to work hand-in-hand to derive data driven value to the busi ness
A semantic layer is a business representation of data. It enables users to discover and access data through selfservice facility without relying on IT, understand the business definition of data (e.g., customer, provider, and products)and its relation ships with other data assets. Even more than that, user could easily start discovering and integrating, on the fly, data from totally different datasets that used different descriptors. Perhaps the greatest achievement of the semantic data layer is to provide different data professionals with easy access to the data needed for their specific roles and tasks. It also provides data lineage and catalogue information about the data assets. The concept of semantic data layer has been in place since the early 1990s. But the significant change in the data landscape due to rise of big data and with increasing push towards data driven digital transfor mation is now making the semantic data layer increasingly important.
Augmented analytics is a key component of the semantic data layer. Augmented analytics uses data science/machine learning, artificial intelligence, and various natural language processing technologies (like natural language generation and natural language query) to enable automation that would accelerate obtaining answers from business data all through a simple, user directed process.
The semantic data layer could be abstracted from the complexities or accessing and querying data from the user by providing a set of Data Service Interfaces (DSI) that could be integrated into business systems whether internal or customer facing and operational processes. With a robust semantic data layer in place, teams can work together more effectively by sharing and collaborating in data value creation, thereby enabling them to derive quality & better insights and make better decisions in less time. Data Science requires close to 80 percent of time in preparing data for value creation, and these overheads can be reduced significantly through the semantic layer. This layer also contributes to managing security and governance of the data assets better. The semantic data layer therefore empowers data processes that democratize data.
•Data Quality:An organization may have the best business and operational processes, the best and state-of-the art technology environment, the smartest brains that can process and analyse data i.e.best data scientists and AI practitioners to generate value, and the best data, technology and digital strategies. But if the underlying data that touches these people, processes and technology is poor in terms of its quality and integrity, the outcomes derived will be poor. Quality of outcomes generated through these initiatives is directly proportional to the quality of data. It is there fore, important that organizations must include 'Data Quality of Design' discipline when planning initiatives at the planning stage itself. Data quality capabilities must include data profiling, cleansing, and enrichment.
•Data Governance:Governing the democratized data, the semantic data layer, the data definitions, data quality, data privacy, data security, data standards, data retention and destruction, and any data related risks is critical. Traditional approaches to data governance where an organization tries to govern all its data assets through a big bang approach rarely works nowadays as businesses neither have the patience nor the budget for it. It is therefore, important to apply smart governance framework by looking at governing the Critical Data Elements that would create value for the business through the use of BI, Data Science and AI, and by incrementally building data governance culture in the organization by demonstrating that data governance enables value creation rather than been seen as controlling or slowing progress.