
Disappearance of the Bad Debts - Building Technology Ecosystem to Create Transparency & Weed-out Bad Cases Early!

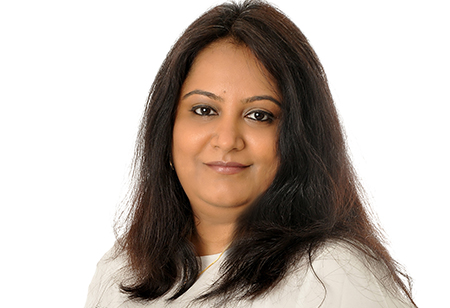
Meghna Suryakumar, Founder & CEO, Crediwatch, 0
India’s formal banking sector has been grappling with bad debts since years, and the broader economy is starting to face the repercussions. Information disclosed by the Reserve Bank of India(RBI) under the Right to Information (RTI) Act, revealed that public sector banks in the country have virtually lost Rs 1.07 lakh crore in bad debts, owing to 88 biggest defaulters. According to central bank data reviewed by Reuters, Indian banks wrote off over $30 billion worth of bad debt in the fiscal year ending on June 30. The write offs indicate the severity of the bad loan crisis in India, which poses a huge threat to the country’s economic growth. In fact, Indian banks now hold the distinction of having the world’s worst bad loan ratio. To add to the misery, the fears of an economic slump have also affected the NFBCs and digital lending platforms. The scenario yet again draws attention to the inefficiency of the traditional credit rating and underwriting methods, and calls for the need for a robust, tech-driven credit rating system. An obvious query that follows is how technological interventions can help lenders mitigate risks and weed out bad cases early on. Advances in the fields of AI, ML and Big Data have made it possible for financial institutions to tap into alternative sources of data that have not been assessed previously and make more informed lending decisions.
Diving Deep into Alternative Data
Alternative data has become the buzzwords of sorts in the financial services industry, and rightly so. With the help of Big Data, lenders can have a comprehensive picture of the prospective borrower, drawing meaningful insights from a vast pool of alternative data sets that may include promoter backgrounds, statutory payment statues, litigation history, defaulter list scans, international trade data, compliance, utility bill payment history and adverse media sentiment to name a few. Underwriting models that combine traditional scores with alternative data can create accurate borrower profiles by improving the accuracy of default predictions. The use of alternative data in credit rating and underwriting presents not only helps financial institutes improve their risk assessment ability, but also provides greater access to credit to the unserved/underserved
segments of small businesses. This can address the massive lending gap that is attributed to credit discrimination. In a nutshell, alternative data presents a win-win situation for both lenders and borrowers.
Automated Decision Making for Quicker Loan Processing
Modern technologies hold great promise for automating and streamlining credit underwriting. Traditionally, financial institutes have been using a manual process for scoring and approving or rejecting loans, wherein a group of analysts score each loan against a set of financial metrics that are in place to determine the borrower’s acceptability. Needless to say, this entire process is not only time-consuming, but also more error-prone. However, a data-driven, automated scoring system is capable of analyzing quantitative and qualitative risk factors to give accurate credit scores in near realtime. This subsequently enables rapid processing and approval loans, resulting in a seamless customer onboarding process. Moreover, an automated scoring system allows credit underwriters to focus on more complex aspects that might require human intervention, like looking into other contingencies that the data may not reveal. While fully automated decision making may not be feasible for complex, highvalue loans, it can certainly help banks and NBFCs increase consistency in their credit underwriting method.
Credit risk Analysis with Machine Learning
The credit-risk analysis essentially refers to assessing the possibility of the borrower’s failure to repay the loans and the loss caused to the financer. When done manually, credit risk assessment can take up to days and runs higher risks of mistakes. This is where machine learning comes to the rescue. ML-based tools have the ability to identify complex, nonlinear patterns within large volumes of data, which paves the way for a more accurate credit-risk analysis. Based on such analysis, lenders can then decide the true credit-worthiness of the borrower and significantly reduce default cases. By incorporating powerful algorithms such as artificial neural networks, random forest and boosting, financial institutes can improve their operational efficiency while addressing the early warning signs.
Given India’s history of bad debts, moving beyond conventional credit models is no longer an option, but a necessity. There is an imminent need for large scale adoption of technologies like AI, ML and Big Data in credit scoring and underwriting process to facilitate better decision making for lenders. After all, technology is the key to solving the critical challenges faced by the Indian banking and finance sector.
A data-driven, automated scoring system is capable of analyzing quantitative and qualitative risk factors to give accurate credit scores in near realtime
Automated Decision Making for Quicker Loan Processing
Modern technologies hold great promise for automating and streamlining credit underwriting. Traditionally, financial institutes have been using a manual process for scoring and approving or rejecting loans, wherein a group of analysts score each loan against a set of financial metrics that are in place to determine the borrower’s acceptability. Needless to say, this entire process is not only time-consuming, but also more error-prone. However, a data-driven, automated scoring system is capable of analyzing quantitative and qualitative risk factors to give accurate credit scores in near realtime. This subsequently enables rapid processing and approval loans, resulting in a seamless customer onboarding process. Moreover, an automated scoring system allows credit underwriters to focus on more complex aspects that might require human intervention, like looking into other contingencies that the data may not reveal. While fully automated decision making may not be feasible for complex, highvalue loans, it can certainly help banks and NBFCs increase consistency in their credit underwriting method.
Credit risk Analysis with Machine Learning
The credit-risk analysis essentially refers to assessing the possibility of the borrower’s failure to repay the loans and the loss caused to the financer. When done manually, credit risk assessment can take up to days and runs higher risks of mistakes. This is where machine learning comes to the rescue. ML-based tools have the ability to identify complex, nonlinear patterns within large volumes of data, which paves the way for a more accurate credit-risk analysis. Based on such analysis, lenders can then decide the true credit-worthiness of the borrower and significantly reduce default cases. By incorporating powerful algorithms such as artificial neural networks, random forest and boosting, financial institutes can improve their operational efficiency while addressing the early warning signs.
Given India’s history of bad debts, moving beyond conventional credit models is no longer an option, but a necessity. There is an imminent need for large scale adoption of technologies like AI, ML and Big Data in credit scoring and underwriting process to facilitate better decision making for lenders. After all, technology is the key to solving the critical challenges faced by the Indian banking and finance sector.