
IIoT for Predictive Maintenance & Process Optimization

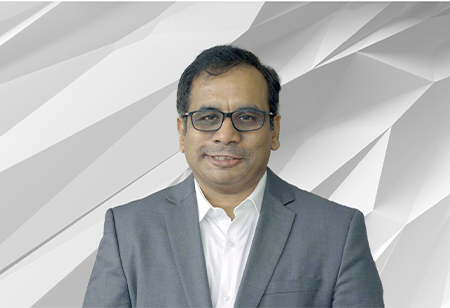
Rajesh Ramachandran, Global Chief Digital Officer & MD, ABB Process Automation, ABB Group, 0
In a recent conversation with Bimlesh Prasad (Correspondent, CEO Insights), Rajesh shared his insights on IIoT and AI and how they both can together significantly improve a manufacturing business to achieve safer, smarter, and sustainable operations. Below are a few select extracts from the exclusive interview –
How is Industrial IoT enhancing the efficiency of enabling predictive maintenance in a manufacturing setup?
Predictive maintenance has always been the topmost critical use case of Industrial IoT, as this is where we see the combination of data, domain expertise, IoT platform, and AI. With a combination of these four critical aspects, predictive maintenance enables manufacturers to predict anomalies in their plants – be it in terms of equipment health, performance, reliability, or operational parameters well in advance. All these aspects of an asset can be determined by analyzing the data that is coming in from various physical sensors and soft sensors (based on systems data), along with its context to model, analyze, and predict. As an underlying scalable platform, IIoT is the backbone for achieving holistic asset performance management, asset strategy, and investment planning. Some of the key aspects of an effective IIoT-based predictive maintenance system are device management, real-time integration capabilities across Operational/Engineering/IT systems, effective data management, cybersecurity, AI & analytics, digital twins, application enablement, and many others.
What is the most critical challenge that organizations face when adopting IIoT for predictive maintenance?
While it depends on the type of industry and assets, the top most challenge has been Data readiness in terms of availability quality, and integrity. While AI is considered to be the core engine of a predictive maintenance system, data is the fuel that keeps the engine running. In other words, the data that is being fed into the AI application for analysis is what drives the efficiency of the predictive maintenance system. For manufacturing companies in particular, the challenge is to ensure the quality and accuracy of data. This is because, if the data that is being gathered through various sensors and systems in an industrial setup is not accurate, the output of the AI
will also be incorrect, leading to an untrustworthy system. Going further, most organizations install IoT devices and sensors across their manufacturing plant but do not pay attention towards the timely repair and maintenance of those sensors. As a result, there is also a risk of inaccurate readings being recorded by those sensors, degrading of the quality of data that is being collected and fed into the predictive maintenance systems. Another challenge equally faced by organizations is the shortcut approach of not leveraging all relevant data to build models. Thanks to AI, where it can also simulate ‘soft sensors’ based on the data patterns of both time series data as well as context from maintenance systems data such as from SAP.
Throw some light on how predictive maintenance can contribute towards cost savings and increased operational efficiency.
IIoT enables companies to unlock 73 percent of the data which is otherwise not used for analysis. IIoT enabled predictive analytics to automate the translation of data into contextual data, which when applied to an AI system, enhances productivity, increases asset life by 20-25 percent, and reduces maintenance costs by 35 percent. All this will in turn result in a significant increase in operational efficiency. By having a proper predictive maintenance strategy along with a scalable IIoT platform, manufacturers can easily cut down their operational costs by 25-30 percent. Augmenting Generative AI capabilities, we see the value creation further improves exponentially.
What is your advice to young industry leaders who aspire implementing IIoT-based predictive maintenance into their operations?
We are at the cusp of maximizing industry 4.0, which is all about data-driven digital processes to optimize the overall operations of the plant. Today, we have the right technologies and easy access to a variety of highly efficient software platforms and applications that are very flexible and easy to deploy across any kind of environment. This software also enables companies to integrate, contextualize, and modeling the data for prediction and optimization use cases. Also, these softwares with the new business models such as SaaS and Hybrid models come at a very reasonable price and do not require huge capital; meaning that even small businesses and startups can leverage them to achieve heightened optimization across their entire process cycle. However, it is paramount for business leaders to identify the right partner to work with in accordance with the readiness of their data and their cybersecurity and data privacy norms. and select the partner who brings the highest value addition to your organization in driving the business outcomes as a co-pilot not just providing software. Furthermore, carrying out a pilot project with the selected vendor is also a very effective and highly recommended move in this regard to reconfirm the readiness of data and processes to scale.
Throw some light on how predictive maintenance can contribute towards cost savings and increased operational efficiency.
IIoT enables companies to unlock 73 percent of the data which is otherwise not used for analysis. IIoT enabled predictive analytics to automate the translation of data into contextual data, which when applied to an AI system, enhances productivity, increases asset life by 20-25 percent, and reduces maintenance costs by 35 percent. All this will in turn result in a significant increase in operational efficiency. By having a proper predictive maintenance strategy along with a scalable IIoT platform, manufacturers can easily cut down their operational costs by 25-30 percent. Augmenting Generative AI capabilities, we see the value creation further improves exponentially.
As an underlying scalable platform, IIoT is the backbone for achieving holistic asset performance management, asset strategy, and investment planning.
What is your advice to young industry leaders who aspire implementing IIoT-based predictive maintenance into their operations?
We are at the cusp of maximizing industry 4.0, which is all about data-driven digital processes to optimize the overall operations of the plant. Today, we have the right technologies and easy access to a variety of highly efficient software platforms and applications that are very flexible and easy to deploy across any kind of environment. This software also enables companies to integrate, contextualize, and modeling the data for prediction and optimization use cases. Also, these softwares with the new business models such as SaaS and Hybrid models come at a very reasonable price and do not require huge capital; meaning that even small businesses and startups can leverage them to achieve heightened optimization across their entire process cycle. However, it is paramount for business leaders to identify the right partner to work with in accordance with the readiness of their data and their cybersecurity and data privacy norms. and select the partner who brings the highest value addition to your organization in driving the business outcomes as a co-pilot not just providing software. Furthermore, carrying out a pilot project with the selected vendor is also a very effective and highly recommended move in this regard to reconfirm the readiness of data and processes to scale.