
Leveraging The Power Of AI-Enabled Predictive Analytics For Moving Beyond Forecasting To Prescriptive Insights

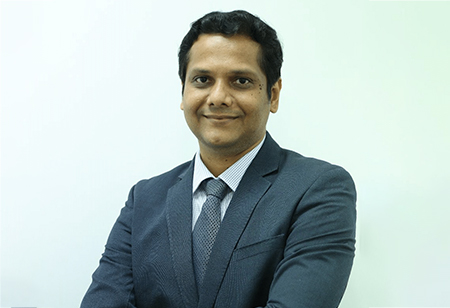
Anurag Sinha, Co-Founder & Managing Director, Wissen Technology, 0
There's a subtle yet exceptionally critical difference between ‘prediction’ and ‘prescription when talking with respect to analytics and extracting valuable insights for decision-making.
Prediction is simply the prediction of a future event or circumstance based on some assessment of the historical data. An example of the same could be a prediction about XYZ percent of consumers not renewing their subscription after the first month.
Prescription, on the other hand, is the act of formulating a course of action that should be taken in certain circumstances. So, for example, prescriptive analytics would bring in suggestions about offering discounts on subscription renewal or driving targeted marketing, etc., to reduce the percentage of consumers who would otherwise not renew the subscription.
So, as we can see, predictive and prescriptive analytics are intertwined. With good predictions in place, companies can move from 'just' forecasting to prescription.
Moving From Forecasting to Prescriptive Insights
As elucidated above, predictive analytics uses historical data to forecast future outcomes. This can be helpful for businesses to make informed decisions about things like demand planning, risk management, and customer churn. However, there's a catch! Predictive analytics can only tell you what is likely to happen. It cannot tell you what you should do about it.
And this is not good for two major reasons:
• You don't have a path to move from insight to action. So, capitalizing on predictions is fairly tough.
• The phenomenon of analysis paralysis can kick in. With so many predictions in place without any clear directive, there's a chance that decision-making will be exceptionally challenging.
Enter Prescriptive Analytics! This approach uses AI to not only predict the future but also recommend the best course of action to take. For example, a retailer could use prescriptive analytics to predict which products are likely to be in high demand. They can then use this information to adjust their inventory levels and marketing campaigns accordingly. This could help them to avoid stock-outs and maximize sales.
Another example is a bank that could use prescriptive analytics to predict which customers are likely to default on their loans. This information could then be put to use to offer those customers financial counseling or other interventions to help them avoid default.
So, how do we consider predictive analytics as a rudimentary base and move beyond forecasts to prescriptions?
Collating the Most Pertinent Data
Because prescription is more advanced than prediction, there's a need for high-quality data at disposal. So, the foundational step should be to collate the most pertinent data.
Identifying Patterns and Trends
Machine learning and statistical models should be used to identify patterns and trends in data that would
otherwise be difficult or impossible to discern. It's here that the nature of the problem (such as time series forecasting or regression) should be defined. This paves the way for model training and validation.
Laying Out Concrete Objectives
What are the decision variables under consideration? What are the objectives of analytics? For example, is the business looking to reduce customer churn, amplify profits, or improve user engagement? This is also where modeling the constraints to keep the prescriptions within realistic bounds becomes critical.
Providing Recommendations
AI can help provide recommendations for actions that can be taken to improve outcomes. For example, AI can recommend which products to promote to a specific customer or how to allocate resources to optimize production.
Optimizing Processes
Prescriptive analytics relies heavily on optimization or rule-based algorithms since these can serve to improve decision-making. Some prominent examples of optimization algorithms include linear programming, integer programming, etc.
Feedback & Refinement
Based on how the prescriptive recommendations perform, it's critical to refine the model and even objectives and constraints.
Challenges of Using AI-Enabled Predictive Analytics
Of course, charting the path from predictions to prescriptions is not without its fair share of challenges. Here's a rundown of the same:
Data Quantity Dilemma
One of the foremost challenges in realizing successful prescriptions is the requirement for vast quantities of data. These systems thrive on large datasets to generate meaningful insights and high-quality predictions. However, it's noteworthy that businesses often struggle to accumulate and maintain the necessary volume of information, making it a critical challenge to address.
Precision Predicament
Another significant challenge is the demand for precise and high-quality data. Prescriptive analytics heavily depends on accurate information to yield reliable results. Inaccurate or outdated data can lead to flawed predictions and unoptimized course of action suggestions.
Consider this: in prescriptive analytics, we're not only focusing on leveraging data to inform predictions. We're also looking for "prescriptive content of data" — i.e., the efficacy of the data at our disposal to inform decisions or courses of action that won't be immensely affected by the uncertainty of decision variables. So, it becomes imperative for organizations to ensure data quality and integrity.
Talent Shortage Troubles
The shortage of skilled professionals well-versed in developing and utilizing AI models poses a substantial challenge. Building and maintaining AI-powered analytics models requires specialized expertise. As such, organizations need to invest in training or hiring qualified personnel to harness the full potential of AI in their operations.
Conclusion
The difference between forecasting and prescriptions comes down to analytics' focus, complexity, and data input. With powerful AI-enabled predictive models in place riding on high-quality data, it's very much possible to create excellent quality prescriptions. However, enterprises must collaborate with experts to ensure that their analytics investments don't go down the drain and, instead, prove viable in charting a successful data-driven growth story. At Wissen, we can help enterprises realize just that. Contact us to learn more.
Laying Out Concrete Objectives
What are the decision variables under consideration? What are the objectives of analytics? For example, is the business looking to reduce customer churn, amplify profits, or improve user engagement? This is also where modeling the constraints to keep the prescriptions within realistic bounds becomes critical.
Providing Recommendations
AI can help provide recommendations for actions that can be taken to improve outcomes. For example, AI can recommend which products to promote to a specific customer or how to allocate resources to optimize production.
Prescriptive analytics relies heavily on optimization or rule-based algorithms since these can serve to improve decision-making.
Optimizing Processes
Prescriptive analytics relies heavily on optimization or rule-based algorithms since these can serve to improve decision-making. Some prominent examples of optimization algorithms include linear programming, integer programming, etc.
Feedback & Refinement
Based on how the prescriptive recommendations perform, it's critical to refine the model and even objectives and constraints.
Challenges of Using AI-Enabled Predictive Analytics
Of course, charting the path from predictions to prescriptions is not without its fair share of challenges. Here's a rundown of the same:
Data Quantity Dilemma
One of the foremost challenges in realizing successful prescriptions is the requirement for vast quantities of data. These systems thrive on large datasets to generate meaningful insights and high-quality predictions. However, it's noteworthy that businesses often struggle to accumulate and maintain the necessary volume of information, making it a critical challenge to address.
Precision Predicament
Another significant challenge is the demand for precise and high-quality data. Prescriptive analytics heavily depends on accurate information to yield reliable results. Inaccurate or outdated data can lead to flawed predictions and unoptimized course of action suggestions.
Consider this: in prescriptive analytics, we're not only focusing on leveraging data to inform predictions. We're also looking for "prescriptive content of data" — i.e., the efficacy of the data at our disposal to inform decisions or courses of action that won't be immensely affected by the uncertainty of decision variables. So, it becomes imperative for organizations to ensure data quality and integrity.
Talent Shortage Troubles
The shortage of skilled professionals well-versed in developing and utilizing AI models poses a substantial challenge. Building and maintaining AI-powered analytics models requires specialized expertise. As such, organizations need to invest in training or hiring qualified personnel to harness the full potential of AI in their operations.
Conclusion
The difference between forecasting and prescriptions comes down to analytics' focus, complexity, and data input. With powerful AI-enabled predictive models in place riding on high-quality data, it's very much possible to create excellent quality prescriptions. However, enterprises must collaborate with experts to ensure that their analytics investments don't go down the drain and, instead, prove viable in charting a successful data-driven growth story. At Wissen, we can help enterprises realize just that. Contact us to learn more.