
Threat Detection & Prevention through Deep Learning

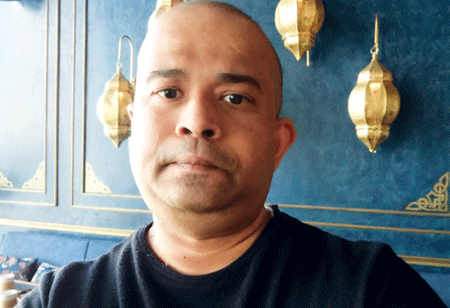
Vinay Lohar, Cybersecurity Manager, Siemens, 0
The pace and complexity at which cyber attacks are expected by the beginning of the year 2020 will make organizations rethink their strategy and deploy cutting edge technology for effective threat detection. Detection of threats before they cause damage is therefore crucial for all organizations. But this capability is not always what it should be. In several cases, the average time it took for an organization to realize it had been breached was 60 days. This time is more than enough time for significant damage to take place.
Advanced threat detection is not possible without using technologies like Artificial Intelligence(AI) and its subsets like Deep Learning(DL), User & Entity Behaviour Analytics and Intelligent Big Data Analysis. Today, organizations need to take care of seven stages of cyberattack kill chain to best protect themselves. These are reconnaissance, initial compromise command and control, lateral changes target attainment and finally, exfiltration, corruption & disruption and above all the weak human link.
Hackers are no more individuals who use a system to launch one or two attacks at a time. Today hackers can launch millions of attacks and spread malware quickly across the network. This makes rapid threat detection of threats with the help of AI increasingly important capability before they cause damage. AI based threat detection systems will have a complex role in a world where every second device would soon be an Internet of Things (IoT) based device.
In order to be effective, these threat detection systems need to know the types of roles people in an organization have, what kind of access rights do they have and how safely does the organization manage data. These are only the initial steps. What precedes before planning the next course of action is countless hours of hard work to understand, internal workflows, data assets, technology, processes, roles and responsibilities. DL can take into account, the setup needed for effective threat detection, for example by mapping user accounts (e.g. local storage VPN, emails, cloud storage) and related identifiers(user, name, email address, business division team and so on) to individual user identities to create baselines of behaviour. By associating well crafted modules and algorithms to study user behaviour, all relevant user activity will be accounted for during analysis. These baselines can be profiled against a
historical baseline of that user’s activity, as well as those of the user’s peers. By doing so, the DL module will initiate threat detection and prevention process.
Even today the worst threats are the ones that are seldom detected. This means threat detection systems need to be good at detecting previously unknown threats. This is extremely important in a world where, threats are increasing in number and complexity, while most of the organizations struggle with staffing shortages lack of required skillsets, attrition, false alarms and inefficient rather outdated workflows. AI can recognize significant changes in user behaviour that suggest a security risk but these could also be false positives or internal automations that malfunction. Therefore advanced deep learning modules must be deployed to detect such threats. All deep learning algorithms must keep evolving. We all know that there is nothing called field proven threat model which can support indepth analytics to detect known and unknown threats improve prioritization of issues and support further investigation. Technology that looks cutting-edge today could well become obsolete tomorrow. The future of Deep Learning in AI is all about upgrades and continuous learning. The best part about some of these deep learning modules is that these modules learn from the environment to protect against current and future threats, and continuously evolve without manual intervention. This is what we expect.
AI in threat detection will also change the role of security analysts in security operations centers. These analysts will be able to offload time consuming tasks to focus on important problems that require their expertise, while better analytics are used to discover the threats that may otherwise go unnoticed. The role of humans in Cyber forensics will change. Humans will slowly work as assessors instead of investigators who once took months to discover what exactly happened.
But this is not the only factor. Cybersecurity Awareness for employees will play a critical role in maintaining a safe work environment. What technicians see as a user with privileged access uploading sensitive information to cloud storage could be an automated malware or compromised credentials. With a growing proportion of attacks conducted via compromised credentials, even the most sophisticated anti-virus solutions fail to work. Thus every organization needs 'supervised learning modules’ in form of Cybersecurity Awareness Sessions, Web Based Trainings, Cybersecurity Conferences and Gamification which is currently being managed by quite a big list of organizations. Whether a new strain of ransomware or an emerging insider attack, the Enterprise Immune System cannot function without Awareness. This Cybersecurity awareness will assist all DL based threat detection modules.
AI Based Threat Detection Systems Will Have A Complex Role In A World Where Every Second Device Would Soon Be An Internet Of Things(IoT )Based Device
Even today the worst threats are the ones that are seldom detected. This means threat detection systems need to be good at detecting previously unknown threats. This is extremely important in a world where, threats are increasing in number and complexity, while most of the organizations struggle with staffing shortages lack of required skillsets, attrition, false alarms and inefficient rather outdated workflows. AI can recognize significant changes in user behaviour that suggest a security risk but these could also be false positives or internal automations that malfunction. Therefore advanced deep learning modules must be deployed to detect such threats. All deep learning algorithms must keep evolving. We all know that there is nothing called field proven threat model which can support indepth analytics to detect known and unknown threats improve prioritization of issues and support further investigation. Technology that looks cutting-edge today could well become obsolete tomorrow. The future of Deep Learning in AI is all about upgrades and continuous learning. The best part about some of these deep learning modules is that these modules learn from the environment to protect against current and future threats, and continuously evolve without manual intervention. This is what we expect.
AI in threat detection will also change the role of security analysts in security operations centers. These analysts will be able to offload time consuming tasks to focus on important problems that require their expertise, while better analytics are used to discover the threats that may otherwise go unnoticed. The role of humans in Cyber forensics will change. Humans will slowly work as assessors instead of investigators who once took months to discover what exactly happened.
But this is not the only factor. Cybersecurity Awareness for employees will play a critical role in maintaining a safe work environment. What technicians see as a user with privileged access uploading sensitive information to cloud storage could be an automated malware or compromised credentials. With a growing proportion of attacks conducted via compromised credentials, even the most sophisticated anti-virus solutions fail to work. Thus every organization needs 'supervised learning modules’ in form of Cybersecurity Awareness Sessions, Web Based Trainings, Cybersecurity Conferences and Gamification which is currently being managed by quite a big list of organizations. Whether a new strain of ransomware or an emerging insider attack, the Enterprise Immune System cannot function without Awareness. This Cybersecurity awareness will assist all DL based threat detection modules.