
Generative AI Demystified: A CEO's Handbook for Success

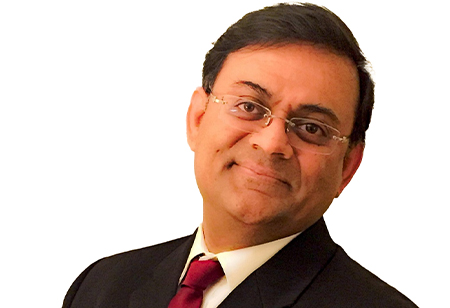
Amit Gautam, Co-founder and CEO, Innover, 0
The journey will commence with a fundamental question: do the chosen solutions align with the company's overarching strategic goals and long-term vision? This initial decision will set the course. Subsequently, organizations will have to conduct a thorough evaluation of critical factors such as budget, data scale and quality, privacy and security requirements, latency, and request volume. The optimal approach will also depend on a company's aspirations and willingness to tolerate risks. Once organizations identify their golden use cases, they will need to make strategic choices regarding whether to fine-tune existing Large language Models (LLMs) or train a custom model to attain desired outcomes.
The Cross-Roads: Off-the-Shelf Models, Building a Custom Model, or Fine-Tuning?
Every business is unique, with its own operational nuances, industry insights, and historical data that cannot be fully captured by a one-size-fits-all model. With a multitude of LLM models available in the market today, organizations face the challenge of selecting the most suitable model for their needs. Many organizations begin by leveraging ‘off-the-shelf’ models, commonly referred to as foundation models. While these generic models offer a wide array of capabilities, they often fall short when it comes to meeting distinct demands of industries. This means CEOs need to understand that these models cannot fully comprehend the unique essence of their business, potentially resulting in sub-optimal performance and limiting customer experiences. Essentially, what is gained over speed and simplicity is often lost in control and customization.
On the other hand, organizations have the option to build their own custom models, trained exclusively with their own data, giving them complete control. However, developing a proprietary LLM is a demanding and resource-intensive undertaking entailing substantial investments regarding time, capital, and expertise. The costs of building and maintaining such models might prove impractical for most organizations, especially in the initial stages of AI adoption.
Given the challenges and constraints around developing custom LLMs, fine-tuning might be a recommended approach to maximize the value and impact of AI models. Through fine-tuning, organizations can harness their existing domain-specific data and augment it with the capabilities of the foundation model – unlocking new performance frontiers. Fine-tuning provides businesses with greater flexibility when it comes to selecting and improving models. It also enables the ability to switch models based on continuous monitoring and evaluation. This way CEOs can strike the right balance between customization and efficiency, whilst maintaining control and cost-effectiveness.
In the future, companies that have the capability to fine-tune foundational AI models within the context of their unique ecosystem will achieve the highest levels of differentiation and maximize their return on investment.
Minimizing Risks, Maximizing Value
To truly realize the value of Generative AI initiatives, organizations need to mitigate a wide array of risks. One primary concern revolves around ensuring the privacy of customer-shared data. When dealing with closed-source LLMs, data is transmitted over the internet to their servers for inference purposes. Ensuring that this data remains confidential and is not utilized for training purposes without proper authorization is crucial. Obtaining clear and explicit consent from customers regarding data usage policies is of utmost importance in this context.
Another crucial piece of advice for CEOs pertains to the
ethical dimensions. Four fundamental dimensions demand their attention: privacy, fairness, bias and robustness. It is not just about preempting potential biases or discriminatory outcomes but also ensuring the reliability of their AI models. For instance, if a LLM consistently suggests higher-priced products to customers based on their specific income level, it can lead to allegations of discrimination, harming the company's reputation and triggering legal consequences. This necessitates using high-quality data and rigorous testing to enhance the efficacy of AI systems.
To overcome these challenges, a rigorous human review process is required to identify and rectify any biased, incorrect, or unjust content before it reaches customers or stakeholders. Through proactive risk mitigation, organizations can maintain a commitment to fairness, instill trust, and ensure that the content generated by their LLM aligns with their intended policies and goals.
Overall, CEOs must bear the responsibility of establishing resilient frameworks to analyze and select the right models, effectively address the risks inherent in their Generative AI adoption journey, and devise use cases that yield value.
Building a Framework to Put Use Cases in Action
While the deployment of Generative AI for solving business use cases remains in its infancy, organizations are taking deliberate steps to prepare for its adoption, recognizing its potential to effectively tackle a range of challenges and substantially elevate productivity. There are three broad categories that fall under the umbrella of Generative AI:
#1 On-Demand Q&A Capabilities:
By leveraging Generative AI models, businesses can build question-answering chatbots and intelligent control towers that offer self-service capabilities to stakeholders. Leveraging these bots, users can seamlessly search, summarize, and retrieve information from bulky documents, extracting actionable insights within seconds. The objective of these solutions is to streamline procedures and minimize the time spent by subject matter experts on repetitive tasks.
#2 Real-Time, Hyper-Personalized Transactions:
The synergy of Generative AI and Machine Learning models promises to enhance personalization, real-time access to information, and overall engagement experiences for customers, suppliers, and employees. These capabilities can be applied to build use cases around domains such as service desk, field services, supply chain visibility, marketing and eCommerce to elevate transaction experience across touch points.
#3 Intelligent Ecosystem & Autonomous Decision Making:
The complete maturity of Generative AI will be realized when it integrates with technologies like voice assistants, speech-to-text, and robotic process automation. This integration will enable real-time impact by acting as an intelligent and independent decision-making system. Generative AI will then have the capability to automate end-to-end transactions with both customers and employees. With its autonomous ability to generate meaningful actions and make decisions based on value, risk, and likelihood, it will fortify business processes.
The Bottom Line
CEOs must take proactive steps to commence their Generative AI journey and harness its promising potential at an early stage. They must carefully evaluate the timing of ambitious investments, weighing the potential costs of moving too quickly on a complex project when the talent and technology may not be fully ready, against the risks of lagging behind in the industry. By taking calculated steps and considering the long-term implications, they can make informed decisions to maximize the benefits of adopting Generative AI. Additionally, they must exercise caution to steer clear of the potential trap known as 'technological purgatory' – a stage where costs escalate, risks amplify, and outcomes stagnate. To safeguard against this risk, it becomes essential to build a robust talent repository equipped to subject Generative AI models to rigorous testing, continuously fine-tuning and enhancing them. This approach ensures that organizations stay on their intended path of progress and innovation.
Companies that have the capability to fine-tune foundational AI models within the context of their unique ecosystem will achieve the highest levels of differentiation and maximize their return on investment.
To overcome these challenges, a rigorous human review process is required to identify and rectify any biased, incorrect, or unjust content before it reaches customers or stakeholders. Through proactive risk mitigation, organizations can maintain a commitment to fairness, instill trust, and ensure that the content generated by their LLM aligns with their intended policies and goals.
Overall, CEOs must bear the responsibility of establishing resilient frameworks to analyze and select the right models, effectively address the risks inherent in their Generative AI adoption journey, and devise use cases that yield value.
Building a Framework to Put Use Cases in Action
While the deployment of Generative AI for solving business use cases remains in its infancy, organizations are taking deliberate steps to prepare for its adoption, recognizing its potential to effectively tackle a range of challenges and substantially elevate productivity. There are three broad categories that fall under the umbrella of Generative AI:
#1 On-Demand Q&A Capabilities:
By leveraging Generative AI models, businesses can build question-answering chatbots and intelligent control towers that offer self-service capabilities to stakeholders. Leveraging these bots, users can seamlessly search, summarize, and retrieve information from bulky documents, extracting actionable insights within seconds. The objective of these solutions is to streamline procedures and minimize the time spent by subject matter experts on repetitive tasks.
#2 Real-Time, Hyper-Personalized Transactions:
The synergy of Generative AI and Machine Learning models promises to enhance personalization, real-time access to information, and overall engagement experiences for customers, suppliers, and employees. These capabilities can be applied to build use cases around domains such as service desk, field services, supply chain visibility, marketing and eCommerce to elevate transaction experience across touch points.
#3 Intelligent Ecosystem & Autonomous Decision Making:
The complete maturity of Generative AI will be realized when it integrates with technologies like voice assistants, speech-to-text, and robotic process automation. This integration will enable real-time impact by acting as an intelligent and independent decision-making system. Generative AI will then have the capability to automate end-to-end transactions with both customers and employees. With its autonomous ability to generate meaningful actions and make decisions based on value, risk, and likelihood, it will fortify business processes.
The Bottom Line
CEOs must take proactive steps to commence their Generative AI journey and harness its promising potential at an early stage. They must carefully evaluate the timing of ambitious investments, weighing the potential costs of moving too quickly on a complex project when the talent and technology may not be fully ready, against the risks of lagging behind in the industry. By taking calculated steps and considering the long-term implications, they can make informed decisions to maximize the benefits of adopting Generative AI. Additionally, they must exercise caution to steer clear of the potential trap known as 'technological purgatory' – a stage where costs escalate, risks amplify, and outcomes stagnate. To safeguard against this risk, it becomes essential to build a robust talent repository equipped to subject Generative AI models to rigorous testing, continuously fine-tuning and enhancing them. This approach ensures that organizations stay on their intended path of progress and innovation.