
Technologically Cognitive Decision-Making Goes Beyond Processing Data

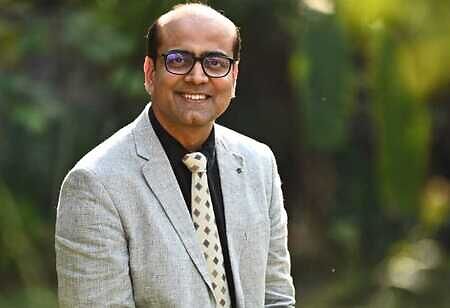
Sarajit Jha, Chief Business Transformation & Digital Solutions, Tata Steel, 0
The method of cognition in the decision-making process is what is undergoing a transformation today, thanks to rapidly evolving digital technologies such as Industrial Internet of Things (IOT), Smart Sensors, and Artificial Intelligence etc.
Earlier, methods were restricted by their capability to Collect, Communicate, Store, and Process the data. As a result, more time expended in collecting and collating data rather than in analysis and decision making.
Today data collection is possible near real-time. The result has been an explosion in all 3 Vs – Volume, Variety, Velocity – of data. From information scarcity, enterprises are at a stage where data is available in abundance.
As a result, the MIS, a key component in any organization’s decision-making process, is now facing a different challenge, that is, making available good quality data to deliver actionable insights.
This leaves the organization with a robust IT Architecture, which becomes a pre-requisite to enable seamless Data Collection, and Virtualization, driving timely insight generation for relevant stakeholders.
A robust and resilient network, ably supported by a strong cyber-security framework with underlying Cloud Infra platforms ensures the availability of data.
This enables sensors, as well as, Level 1 & Level 2 systems to sit on top of the network to collect and transmit the machinery and process data. Parallely, the business process data is collected through transactions in the applications layer. The Cloud infra enables requisite applications to run on top of the data, thereby enabling anytime-anywhere-anyplace compute.
For example, in a Steel plant, a robust manufacturing execution system set-up on the base infrastructure will have functionalities to run the steel making, casting and rolling processes – planning and scheduling, operations management, metallurgical control.
These modules send the order information to the Level 2 process control systems for configuring the next production cycle. The Level 2 data is used for reporting all production data such as Production Trends, Casting Speed, Slab Thickness & Width, Coil weight etc.
Tight integration with costing allows management to have a view on the cost that the company is incurring with every production run. Cost sheets can show material level drill down under each process head from RM till production along with By-product generation for a particular material or plant. Clubbed with visibility on inventory ageing costs, it can form a crucial input into marketing related decisions thereby enabling better working capital management through the delivery of the right information to the right person at the right time.
There are various other examples that highlight how information capture & delivery is being automated. These examples show the availability of quality data is a pre-requisite. Without quality data, decision making is adversely affected. Keeping a tight check on its data quality therefore should be of utmost importance.
Of the eight parameters of assessing Data Quality - Relevance, Usability, Availability, Latency, Uniqueness, Correctness, Accuracy, Security - a robust infrastructure layer helps ensure that the data is available at the relevant frequency, correct (sensor health, system checks etc.), and secure (firewalls, cybersecurity protocols etc.).
Once data availability is ensured, the lens must move to processing the data to make it useful for consumption. Responsibility here lies more with the business teams rather than the IT team. The business team must start by defining what KPIs1 would be of relevance and by making sure that there is a business glossary with a universally agreed definition of the KPIs across the organization. This ensures that everyone in the organization is on the same page with respect to the metrics.
The erstwhile traditional approaches would connect the consumption layers with the source layers to serve ad-hoc data requests. However, problems of scale, speed, and lack of interconnectedness of datasets would emerge soon after as the organisation grew. Today, and going forward, a data lake, supported by a data dictionary and a data catalogue, solves these problems by setting up the platform on top of which analytics can be built by combining data across multiple data sets.
Organizations must constantly evaluate the 4 pillars - Data, Technology, People, Process - that prop-up the high-quality data to ensure that its L3 automation efforts sustain on its journey from merely managing internal tactical needs to be able to seamlessly integrate with ecosystem data and take decisions with high degrees of certainty.
As organizations become data mature, there is a marked reduction in the time being spent in gathering requirements, analyzing, and preparing data. The characteristics of MISs, however, continue to be the same. They need to be timely, comprehensive, and insightful and thereby lead to meaningful action. It is the technology available to deliver the first two which has undergone a step change over the last few decades. MISs are transitioning from being a high-data gathering low-action intensity item to a low-data gathering high-action intensity item. A maturing organisation looking to deliver excellence at all touchpoints, then must transition their IT architecture on similar lines. (Ref: Figure 2). While the base layers of IT Infrastructure & Enterprise Applications continue to form the bedrock of data generation, it is the insights generation through Data & Analytics that needs to be embedded at all user touchpoints.
With the rapidly rising maturity of Machine Learning techniques & technologies, the quality of insights that MISs can deliver is going up – moving from showcasing what has happened to showing a real-time snapshot (Descriptive Analytics via Visualization) to predicting the future with certainty (Prediction). However, it remains a data-driven but human-centric action model subject to the biases of the decision maker.
The next step change involves moving to a scenario where the models aid human beings in taking decisions by suggesting the best course of action (through Simulation & Optimization via Prescriptive Analytics).
The journey eventually culminates in a stage where the machine becomes self-learning & can take actions by itself (Cognitive Analytics/AI2). The intelligent enterprise of tomorrow will be defined by its ability to deploy cognitive analytics extensively across its value chain and thereby build a responsive and agile organization.
The intelligent enterprise of tomorrow will be defined by its ability to deploy cognitive analytics extensively across its value chain and thereby build a responsive and agile organization
Once data availability is ensured, the lens must move to processing the data to make it useful for consumption. Responsibility here lies more with the business teams rather than the IT team. The business team must start by defining what KPIs1 would be of relevance and by making sure that there is a business glossary with a universally agreed definition of the KPIs across the organization. This ensures that everyone in the organization is on the same page with respect to the metrics.
The erstwhile traditional approaches would connect the consumption layers with the source layers to serve ad-hoc data requests. However, problems of scale, speed, and lack of interconnectedness of datasets would emerge soon after as the organisation grew. Today, and going forward, a data lake, supported by a data dictionary and a data catalogue, solves these problems by setting up the platform on top of which analytics can be built by combining data across multiple data sets.
Organizations must constantly evaluate the 4 pillars - Data, Technology, People, Process - that prop-up the high-quality data to ensure that its L3 automation efforts sustain on its journey from merely managing internal tactical needs to be able to seamlessly integrate with ecosystem data and take decisions with high degrees of certainty.
As organizations become data mature, there is a marked reduction in the time being spent in gathering requirements, analyzing, and preparing data. The characteristics of MISs, however, continue to be the same. They need to be timely, comprehensive, and insightful and thereby lead to meaningful action. It is the technology available to deliver the first two which has undergone a step change over the last few decades. MISs are transitioning from being a high-data gathering low-action intensity item to a low-data gathering high-action intensity item. A maturing organisation looking to deliver excellence at all touchpoints, then must transition their IT architecture on similar lines. (Ref: Figure 2). While the base layers of IT Infrastructure & Enterprise Applications continue to form the bedrock of data generation, it is the insights generation through Data & Analytics that needs to be embedded at all user touchpoints.
With the rapidly rising maturity of Machine Learning techniques & technologies, the quality of insights that MISs can deliver is going up – moving from showcasing what has happened to showing a real-time snapshot (Descriptive Analytics via Visualization) to predicting the future with certainty (Prediction). However, it remains a data-driven but human-centric action model subject to the biases of the decision maker.
The next step change involves moving to a scenario where the models aid human beings in taking decisions by suggesting the best course of action (through Simulation & Optimization via Prescriptive Analytics).
The journey eventually culminates in a stage where the machine becomes self-learning & can take actions by itself (Cognitive Analytics/AI2). The intelligent enterprise of tomorrow will be defined by its ability to deploy cognitive analytics extensively across its value chain and thereby build a responsive and agile organization.